CSI Seminar 2015-01-28
From LRDE
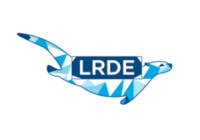
OLENA
11h00 Improving OCR k-NN classifier's training set – Anthony Seure
One part of an OCR toolchain is to classify detected characters: they can be lowercase or capital letters, or digits. To do so, our OCR computes for each image of character an associated wavelet-based descriptor. This descriptor can then be classified. The classification step is currently based on a multiclass k-NN classifier. Since the testing step heavily depends on the number of samples of the training set, the latter can be modified to improve the scores. Our work is focused on the possible improvements of the training set.
SPEAKER ID
11h30 Speaker specific i-vector channel compensation in speaker recognition – Jean-Luc Bounthong
The i-vector is actually the state of the art in speaker verification. Efficient result was achieved using classifier such as Cosine Distance (CD). Howeverclassification is performed on a global channel compensated i-vector. In this study, we explore the possibility to enroll a speaker and define a speaker specific channel compensation using i-vector. The objective is to improve the classifier performance using our previous work on Self-Organizing Map to select suitable i-vector. We will compare the performance of our solution with the global channel compensated method.
12h00 Local channel compensated method in Speaker Recognition System – Jimmy Yeh
Currently, i-vectors become the standard representation of speech context in speaker and language recognition method. Cosine Distance (CD) is the most popular scoring method. It uses Linear Discriminant Analysis (LDA) and Within Class Covariance Normalization (WCCN) to reduce the channel variabilities. The aim of this work is to reduce channel variabilities locally before applying the CD. The idea is to create a large i-vector graph from a training dataset. After clustering it with community detection algorithmsthe target and the test i-vectors are projected into this graph. Only their neighborhood are selected to train the LDA and WCCN. Results will be compared with the global channel compensated method.